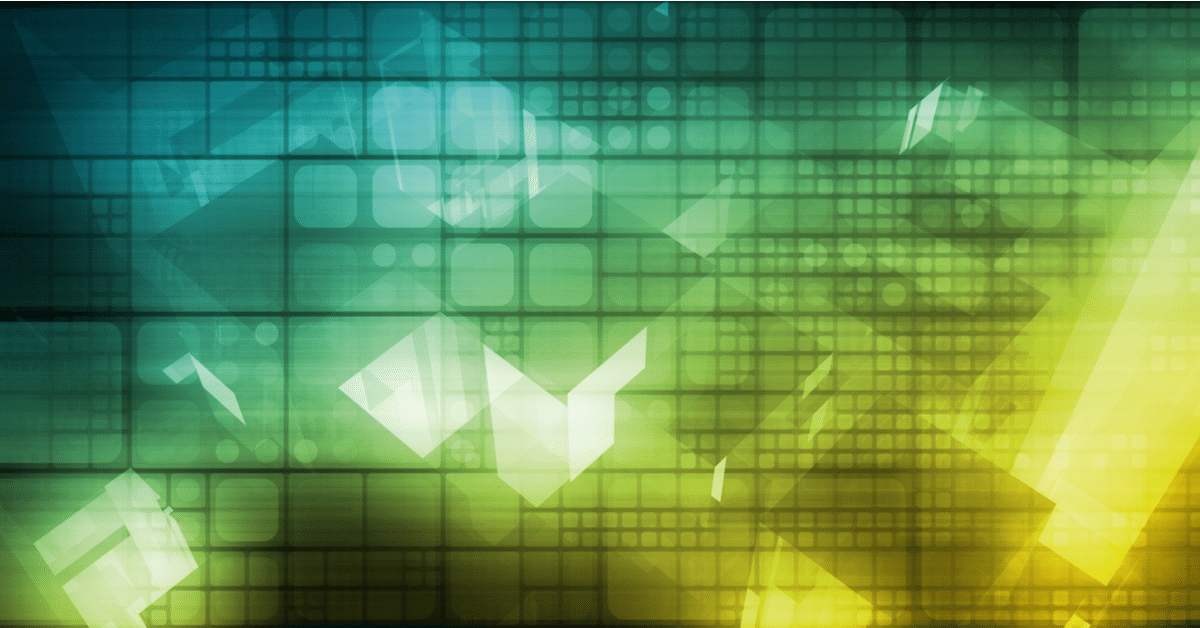
Institutional Knowledge and the Art of Iterative Improvement
Five years into the journey of automating Nuix data processing has delivered some unexpected lessons – among, the most interesting has been the second-order effects.
For example, one of our clients has seen an enormous increase in the number of new Cases they’re creating, but it’s okay because their speed-to-review has also dropped from an average of 5 days to under 10 hours for the first tranche of data processed for each Case.
This success story on the processing side needs to be accompanied by an equivalent improvement on the review side, which will be seeing both more Cases and have less time to get prepared to start the review. Teams with strong communication between functions can make resourcing decisions to manage this surge in the near-term and re-balance resources in the long run.
Or, another client that has become so confident in the quality of their data processing output that they’re starting to process “dark data” pools during downtime and looking for indicators of ROT (redundant, out of date, or trivial data).
Great! Progress on a hard-to-crack risk area, but this progress requires a subsequent process (be it manual, semi- or fully-automated) to deal with the potential ROT.
As we think about the impact of automation on project management and project managers, these second-order effects are front of mind. They highlight the importance of looking at the complete use case, the ability to manage resources across skillsets, and awareness of who needs to know what, and when. One on hand, automation rewards time spent in the design stage of the project solution—on the other, it can create new bottlenecks by stressing other processes.
So far, this series has explored:
- The big picture shift in demand for project managers;
- The importance of business intelligence to managing projects; and
- Principals of “design thinking.”
This post will pivot a bit, from big picture and strategy to applied tactics. Specifically, some of the ways project managers can leverage automation to build, maintain, and use institutional knowledge to drive change in eDiscovery, digital forensics and information governance teams.
Most organizations are committed, in theory, to continuous improvement. But, caseloads and data-loads are increasing, and there are more new types of projects coming down the pipe. This can make it more burdensome than ever for eDiscovery teams to backup that commitment with real resources.
Too often, this burden falls to Project Managers—the people with a project-specific responsibility for rigorous and consistent observation. But, by leveraging this awareness at scale can reinforce intent and win support from leadership and team members.
We think this is because the principles of project management are the best path forward—particularly when reinforced with good automation.
A Good Set of Metrics to Define the Baseline
Its challenging for a team of 3 or 6 Project Managers to share qualitative and allegorical evidence in a meaningful way. So, establishing a program to measure key indicators so everyone has a consistent understanding of current operating parameters is helpful.
Automation makes this easier, because it allows a level of granular detail and control over exactly what happens in a project. Whether it’s measuring performance by task given variation in hardware resources, or defining “deduplication” as a unified series of steps instead of a button click, automation creates confidence in the circumstances that generated any underlying findings, and you (hopefully) have comprehensive and real-time reporting.
Clear Goals
For most data processing teams, the “goal” of a project is to have it look like the baseline. Or, at least, to have it look like the anticipated variation of the baseline.
This can be particularly challenging for teams that are starting to support new types of projects or are seeing new types of data. Defining that anticipated variation from the baseline often requires upfront exploration – which consumes both staff and compute resources—and a strong understanding of downstream process.
This resource constraint may be relaxed for teams that have invested in automation – when it takes 5 minutes of button clicking to run a load file through to export, rather than 90 minutes, your team has more time for the hard stuff.
Automating data processing also makes it a lot easier to run A/B testing. Teams have a specific place to make incremental changes to the process, the real impact of the change is easy to see in the reporting, and the effort required to run multiple tests is a lot lower.
We think this helps teams define their goals ahead of time.
Clear Roles and Responsibilities
Another way to describe “roles and responsibilities” is “who needs to know specific information so they can make related decisions, and when do they need to know it?”.
As teams support new types of projects, or onboard new clients, understanding this network can be difficult. Or, at least, it requires an upfront investment in onboarding the initiative.
One nice thing about an automated data processing workflow is there’s not a meaningful difference between running 1 search list or 1000. Or between sending a “job started” notification to 5 people or conditional “urgent finding” notifications for low-frequency, high-importance scenarios.
It’s a small thing, but, coupled with role-based access controls, a centralized job-monitoring queue, and real-time metrics, good notification practices go a long way to reinforcing roles and responsibilities.
Strong Change Management
At the end of the day, the changes in eDiscovery, digital forensics, information governance and data privacy are not going away. Higher volumes, more cases, weirder data, more sophisticated counterparties…these are facts of the market, not short-term challenges.
Teams that navigate these challenges will need one of two things—a hero mentality (and we all know how quickly heroes burn out) or an agile process with strong adoption rates.
And automation forces adoption—so, as long as updating your automation protocols is relatively painless, it can be a powerful tool in your change management kit.
So, as you think about how your department is going to leverage automation to handle an increased discovery burden—or, support a new use case—invest in understanding your baseline, be clear about what’s changing, defining how roles will be different (and the same) and be specific about how your operations will evolve.
It might be the difference between actual progress, or just moving the bottleneck.